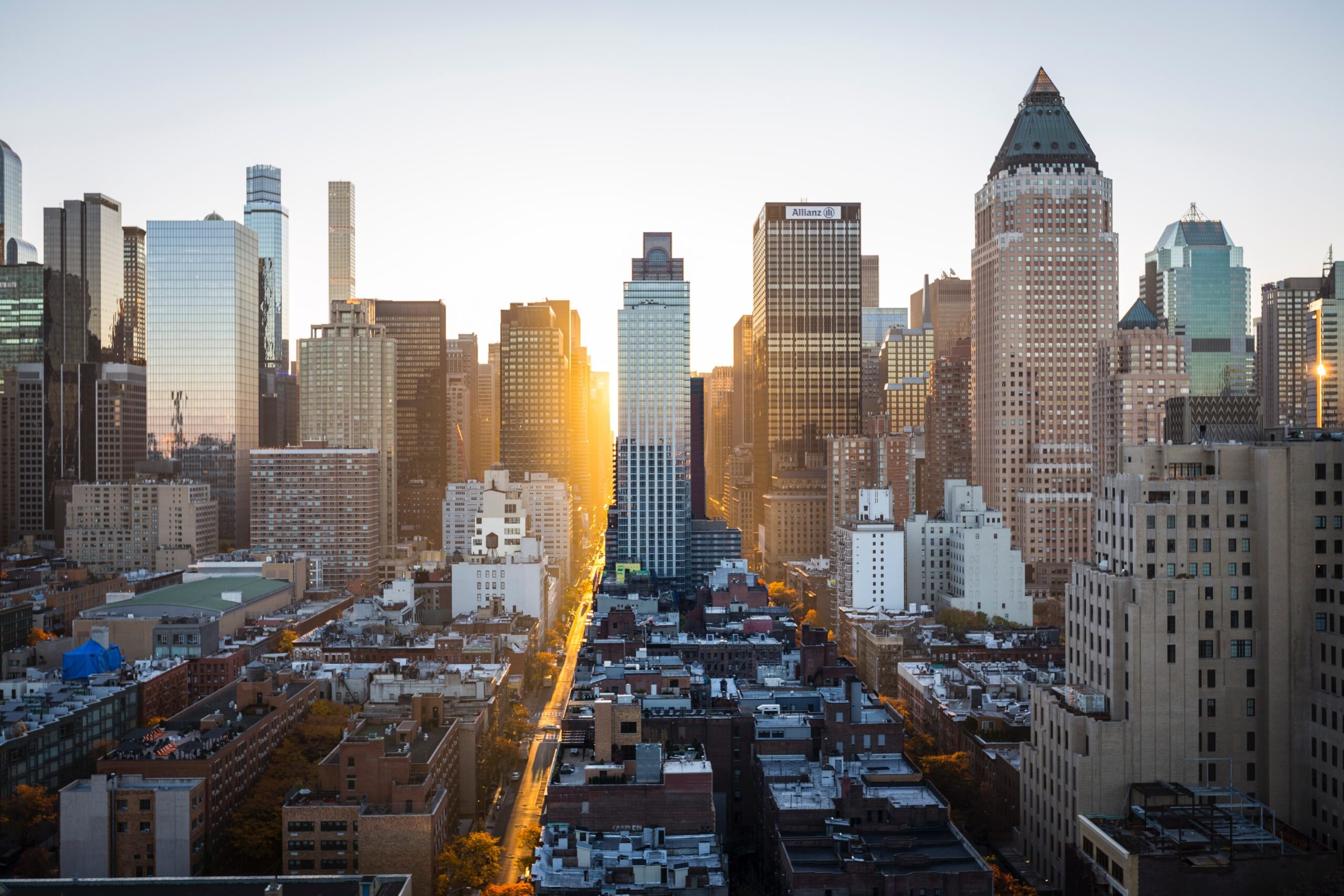
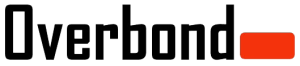
An overload of information and technology can be just as detrimental as having access to too little information and technology; therefore, less is more: the key to greater success is to act with precision. Explore Overbond’s rigorous data aggregation protocol to pull data from numerous sources and extract clean ML-driven insights.
Source: FINANCE MAGNATES
Recent developments in artificial intelligence (AI), web-based communications, and a massive spike in the amount of content that companies and individuals have access to every day has laid the world at our feet; the world has been given the gift of knowledge and information–and the challenge of sorting through it.
And so, investors and investment have been tasked with taking the overwhelming amount of data that they are presented with and making useful tools from it or to risk falling behind the curb.
Haresh Patel, chief executive officer of Mercatus, a data platform for private market investors, told Finance Magnates that “legacy thinking is becoming risky business for financial firms.”
At the same time, however, throwing caution to the wind and simply moving on to the “latest and greatest” piece of investing technology or data is equally risky. The year has started off with a myriad of new tools and sources of data for investors, more advanced and more customizable than ever before–but are investors any better of than they were ten or twenty years ago?
“The software industry built—and firms have bought—departmental solutions to address specific needs, but this has created a maze of data silos which in turn is creating a data disaster,” Patel explained.
Indeed, an overload of information and technology can be just as detrimental as having access to too little information and technology; therefore, less is more: the key to greater success is to act with precision.
But how can investors effectively use the new tools and technologies they have at hand in order to avoid a “data disaster”?
Patel believes that “the most important trend we’re seeing is companies starting to shift away from this legacy thinking and turning their focus instead on creating a data architecture that centralizes and harnesses data across departments, systems, and external sources.”
What does this look like on a practical level?
Patel said that from Mercatus’ perspective “as a company addressing a major pain point for the fastest-growing segment in investing in the financial markets, which is the private market, we see some of the biggest brands using software that is inherently more flexible and enables them to diversify into new asset classes, regions, and unanticipated new business framework.”
These kinds of platforms “harness and centralize internal and external data inputs—from asset operators, energy pricing systems, accounting systems, and more—to facilitate real-time reporting and collaboration across fundraising, investing and asset management teams.”
In other words, “these early adopters are able to connect data, workflows and financial models into a single integrated system powering data-driven decisions, insights, and reports at speed. With advanced analytics and custom dashboards, they are able to quickly gain insight into key trends and KPIs, like performance, risk, forecasts and IRR, all the way down to the asset level.”
The creation of these kinds of tools and platforms has been enabled in part by advances in artificial intelligence (AI) technology. “AI-powered solutions can empower investment firms to make more informed decisions. This includes high precision data analytics: investment patterns, predictive analysis, and client engagement,” explained Victor Fredung, chief executive officer at Shufti Pro, an AI-based identity verification service provider.
“Using machine learning algorithms, investment data can be optimized to enhance portfolio management, as well as operational efficiency. It leads to better reporting, higher accuracy and just better chances of client outreach once decision-making is improved.”
However, AI-powered or not, not all algorithms are created equally–”most algorithms tend to gather ‘all’ of the data rather than just what’s needed,” explained David Bakke, finance expert at personal finance blog Money Crashers.
Bakke believes that, therefore, “It’s pretty much an ‘all or nothing’ type proposition. If you’re going to do this on your own, it will take a lot of time and effort, and you’ll have to be hyper-specific in what you’re searching for. If you’re going to rely on, say, a robo-investing site, there are going to be anomalies that simply can’t be accounted for.”
“So, in a sense, while it might seem like a better strategy than using no data at all, it is a crapshoot.”
However, Bakke does believe that developments in AI could eventually lead to the development of improved algorithms and improved data-gathering systems.
“The AI realm will more than likely see improvements in security and transparency in order to make data more relevant,” Bakke said. “Beyond that, you should see improvements in gathering more intelligent data and the elimination of less necessary data, as this niche continues to improve and expand,” he told Finance Magnates.
At the same time, however, Haresh Patel pointed out that the sudden and sharp rise in the use of AI in data could be in for some big shifts as regulations are built around its usage: “considering that the world is rapidly transitioning to digital, AI will influence nearly every aspect of data, from the way it is collected to the way it is shared and analyzed,” he said. “There will be some major changes coming in 2020.”
However, developments in artificial intelligence have also enabled the creation of new kinds of “alternative data” that can be creatively used to inform investors of relevant trends. While alternative data has been around for some time, the field is continuing to grow in popularity–but, like AI, having access to alternative data isn’t the be-all and end-all for good investment strategy; it must be used wisely.
“[Alternative data] may or may not be helpful for fund managers who are mostly looking for specific trends instead of rough aggregate data,” Haresh Patel said.
Essentially, “the key is to categorize data and build profiles based on geographies, markets and personas. In contrast to big data, alternative data can help tailor customer experience and optimize operations.”
But how can the effectiveness of alternative data be determined? In a more recent call with Finance Magnates, Zak Selbert explained that for example, quant funds (funds that select securities by utilizing the capabilities of advanced quantitative analysis) usually want to know if “a data set, just on its own in a silo, has some sort of predictive value–and the answer is either ‘yes’ or ‘no’.”
Selbert went on to explain that the second question that quant funds usually ask about a data set is “whether or not it adds any value”–in other words, “traditional market factors are easily accessible; so if you strip out the effects of traditional market factors, does a data set still have value?”
At this point, “those that stand out” are “orthogonal”–in other words, “they have no relationship to traditional market factors,” he explained.
Investors also need to take into account the “other kinds of market data, including alternative data” that they have access to–and as the field of alternative data continues to grow, investors have more choices than ever before.
It’s also important to keep in mind that not every piece of data or information is appropriate for work or investments in every sector.
For example, Daniela Andreevska, Marketing Director at Mashvisor, a real estate data analytics company, said that in her industry, “the importance of the use of big data, predictive analytics, and AI is constantly growing when it comes to real estate investing decisions”
“ Investors want to base their investment decisions not only on the past performance of rental properties (traditional analytics) but also on their expected performance in the future (predictive analytics). Of course, this future expected performance is heavily based on trends in past performance as well as trends in the general real estate and housing market.”
David Metzler, chief executive officer of CBD Capital Group, a Venture Capital Group focused on acquiring small to medium cannabidiol (CBD) companies in the US, shared that his company “licensed 40M+ e-commerce data points to create a cohort to compare our prospective acquisitions against.”
“[ …]We [also] used a MOS healthcare study that was created by the Rand Corporation all the major health insurers use,” he added. “This gives us a unique ability to use this data to acquire companies with products that statistically have efficacy on customers and thereby is a proxy for customer repurchase rates and loyalty (if the products work in the eyes of their customers, they will buy again).”
As for the cryptocurrency industry, David Bakke suggested that “what you want to look at in terms of data is profits and losses posted by users (which could be a challenge to find, I might add); sales and purchases (a little bit easier to uncover), and yields.”
With all of that said, you’d really be better off using any one of a number of crypto portfolio tools,” such as TradingView, he added.
Ultimately, though, Haresh Patel said that “good decision making is only as good as the data at hand—as the old adage goes: garbage in, garbage out.”
“With the sheer amount of data available today, it takes a constant, company-wide commitment to data ingestion, cleansing, and quality controls, along with a solid understanding of what data will be most critical in facilitating smart, fast investment decisions.”