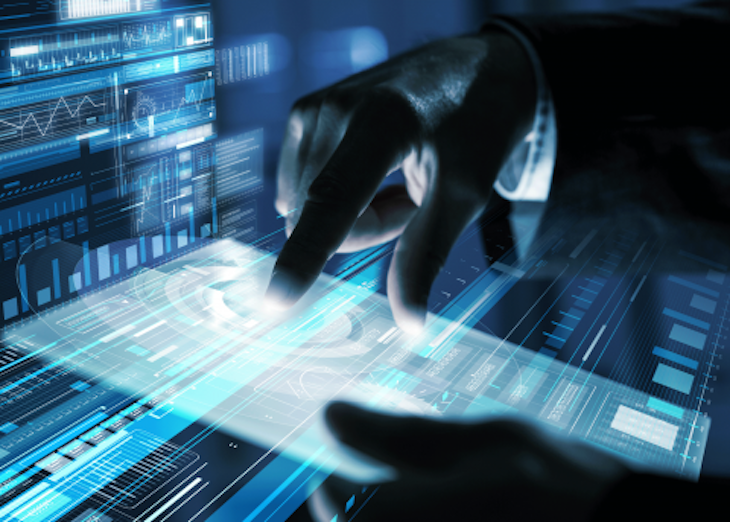
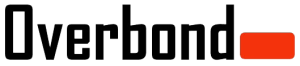
Artificial Intelligence (AI) has been finding its way into the financial services industry offering immeasurable benefits.
Source: The Fintech Times
Artificial Intelligence (AI) has been finding its way into the financial services industry for some time, and there’s no denying that the technology offers immeasurable benefits. AI can increase the efficiency, optimize processes, reduce costs and enrich the customer experience. Possible applications range from customer service and marketing to asset management, portfolio management, treasury, and securities trading.
In areas such as fraud detection, risk management, credit rating and wealth advisory, AI is already augmenting or even replacing human decision makers. In fact, not deploying AI capabilities in these fields can be considered disastrous. With the ever-increasing amounts of data that needs to be processed, AI systems are a must-have to improve accuracy.
As technological capabilities continue to improve, the amount of available data grows, and competitive pressures mount, the use of AI in finance will be pervasive. However, as with any new technology the adoption of AI brings its very own set of challenges.
An AI model is biased when it takes decisions that can be considered as prejudiced against certain segments of the population. One might think that these are rare occurrences – as machines should be less ‘judgmental’ than humans. Unfortunately, as has been proven last year, they tend to be far more commonplace. AI failures can happen to even some of the largest companies in the world. In November 2019, Apple attracted a lot of unwanted social media attention when @DHH, the famous creator of Ruby on Rails accused them of gender discrimination. He and his wife had applied for Apple credit cards together and he received a credit limit 20 times higher than his wife, despite the fact that they file joint taxes, and she had a better credit score. When they approached Apple, the company couldn’t pinpoint the issues driving this result – a clear lack of understanding how the algorithm makes decisions.
How do these biases happen? One reason why algorithms go rogue is that the problem is framed incorrectly. For instance, if an AI system calculating the creditworthiness of a customer is tasked to optimize profits, it could soon get into predatory behavior and look for people with low credit scores to sell subprime loans. This practice may be frowned upon by society and considered unethical, but the AI does not understand such nuances.
Another reason for unintended bias can be the lack of social awareness: The data fed into the system already contains the biases and prejudice that manifests the social system. The machine neither understands these biases nor can it consider removing them, it just tries to optimize the model for the biases in the system.
Finally, the data itself may not be a good representative sample. When there are low samples from certain minority segments, and some of these data points turn out to be bad, the algorithms could make some sweeping generalizations based on the limited data it has. This is not unlike any human decisions influenced by availability heuristics.
Another challenge with regard to AI usage is the question who’s responsible if AI makes a wrong decision. If a self-driving car causes an accident, should it be the fault of the owner who didn’t maintain the car correctly, or did not respond when the algorithm made a bad call? Or is it purely an algorithmic issue? What about our previous example of predatory pricing – within which time frame is the firm employing this algorithm supposed to know that something is amiss and fix it? And to what extent are they responsible for the damages?
These are very important regulatory and ethical issues which need to be addressed. There are risks related to the technology which need to be carefully managed, especially when consumers are affected. This is why it’s important to employ the concept of algorithmic accountability, which revolves around the central tenet that the operators of the algorithm should put in place sufficient controls to make sure the algorithm performs as expected.
An issue often cited when it comes to AI is that many algorithms suffer from a lack of transparency and interpretability, making it difficult to identify how and why they come to particular conclusions. As a result, it can be challenging to identify model bias or discriminatory behavior. It is fair to say that the lack of transparency and the prevalence of black box models is the underlying cause for the two challenges outlined above.
Explainable AI can be a game changer
For financial institutions, it is clear that guidelines need to be put in place to help avoid bias, ensure safety and privacy, and to make the technology accountable and explainable. AI doesn’t have to be a black box – there are ways to make it more intuitive to humans such as Explainable AI (XAI).
XAI is a broad term which covers systems and tools to increase the transparency of the AI decision making process to humans. The major benefit of this approach is that it provides insights into the data, variables and decision points used to make a recommendation. Since 2017, a lot of effort has been put into XAI to solve the black box problem. DARPA has been a pioneer in the effort to create systems which facilitate XAI and it has since gained industry wide as well as academic interest. In the past year, we have seen significant increase in the adoption of XAI, with Google, Microsoft and other large technology players starting to create such systems.
There are still challenges to XAI. The technology is still nascent. And there are concerns that explain ability compromises accuracy, or that adopting XAI compromises the IP of the firm. However, the success of AI will depend on our ability to create trust in the technology and to drive acceptance among users, customers and the broader public. XAI can be a game changer as it will help increase transparency and overcome many of the hurdles that currently prevent its adoption.