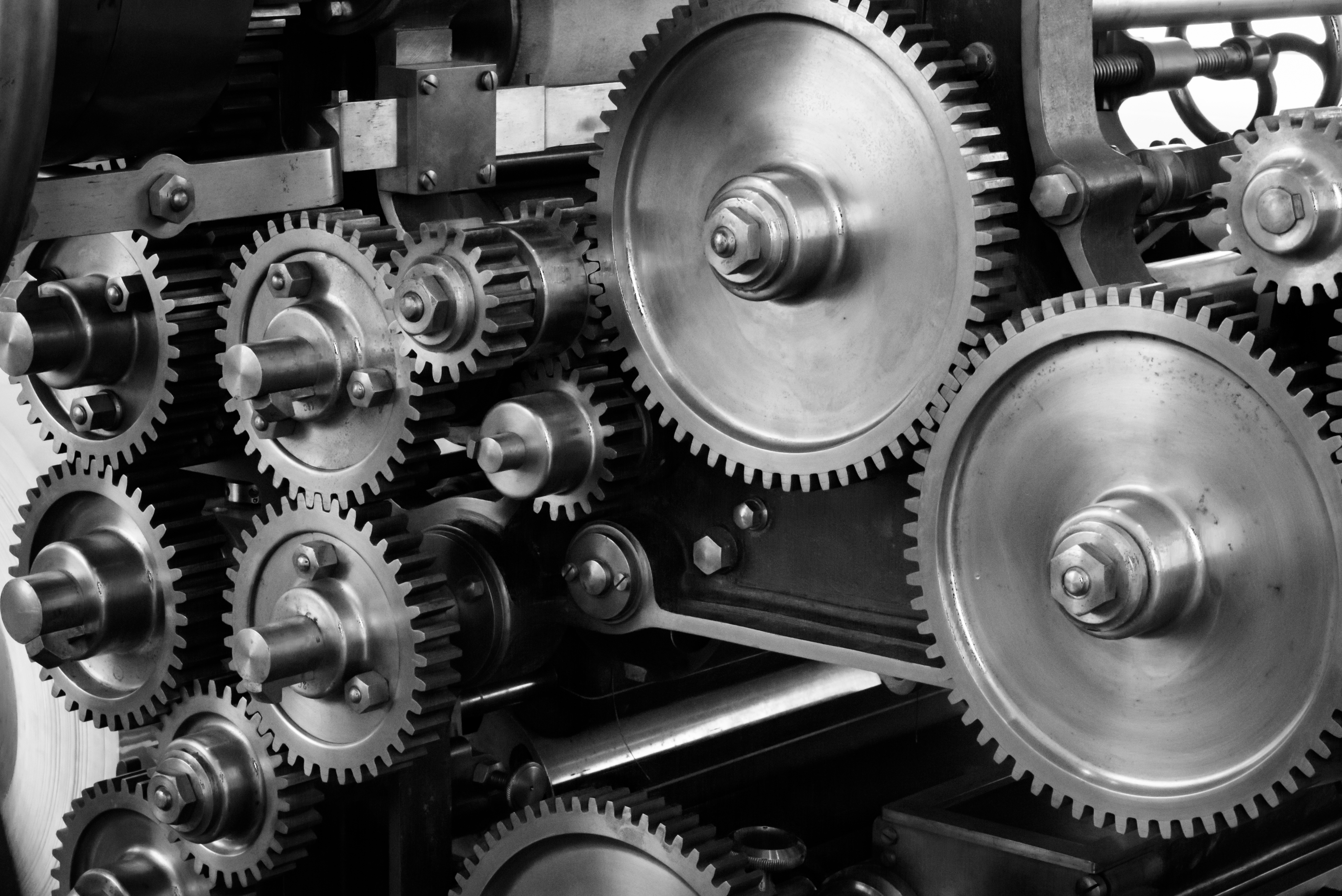
The emergence of big data and machine learning creates an information advantage. This advantage requires building systems that can rapidly integrate disparate data sources.
Source: The Financial Times
Quantitative investment managers always seek to use data in new and innovative ways. The emergence of big data and machine learning are simply an evolution of existing techniques that quantitative investors have long used. However, there are crucial differences between the quant models of the past and the models that can now be created — ones which use artificial intelligence and neural networks to exploit new data sets.
Theoretically, the availability of ever-increasing amounts of information should improve market efficiency — investors today have easy access to vast quantities of company data, news, analysis and commentary, reducing the information gap that may have existed historically and making markets more efficient. However, this does not appear to be the case — markets are just as prone to bouts of irrational fear and exuberance as they have ever been — and this perhaps gives an insight into the practical challenges of working with big data.
Increased amounts of data do not necessarily lead to increased insight unless you are able to process and analyze the information in a timely fashion. Building systems that can rapidly integrate disparate data sources, particularly unstructured data (such as text and natural language), is crucial in creating an information advantage, but this is hard and requires new skills and new investment by market participants.
Even once the information has been gathered it still needs to be analyzed which, given its sheer quantity, has to be done in a systematic and automated fashion. To do this econometric model must be built which identify patterns in the data and trigger investment decisions. Increased processing power and innovations in machine learning means that ever more sophisticated models can be applied, particularly neural networks, to this analysis challenge. As in other fields, such models can learn more and learn faster than human beings are capable of, and ultimately make “better” — more consistent, more reliable — forecasts than any individual could. As a result, these neural network models are a very powerful addition to an investor’s decision-making toolset.
However, while these models are extremely powerful they are, by construction, opaque in nature — the eponymous “black box”. And therein lies the philosophical challenge that we face: how do we engender trust in such models? How do we ensure that as asset managers we fulfil our fiduciary duties as stewards of clients’ assets and truly own and explain our investment decisions?
This is not a new challenge for quantitative investors who have always sought to explain how models work; to lift the hood and show the inner workings. With traditional models you can describe the input data and show its connection to the outcomes — higher future earnings lead to higher valuations, higher debt servicing costs increase the risk of distress. New machine-learnt models capture similar types of relationships but with a much greater degree of subtlety — the relationships are often non-linear and more nuanced; less obvious to the human observer. One advantage of this nuance and subtlety is that individual models are more distinctive, and thus less prone to “crowding” risk than their more traditional counterparts. The downside is that they are that much more complex and less easy to understand.
As a result, asset managers have to invest even more effort in explaining their models when choosing to implement AI and machine learning in their portfolios. This can be done through building visualization tools that illustrate what the models are doing. Proxy (or “white box”) models can be used to open up and learn what the black boxes are doing. Most crucially, asset managers need to ensure their models are grounded in real fundamental insights and principles — first and foremost they need to make sound economic sense.
Building the latest and greatest machine-learnt investment models is only half the challenge. Asset managers need to invest at least as much time and energy in analyzing, validating and ultimately explaining how their models work, as they spent building them in the first place.